New Work Presented at 2021 AMIA Informatics Summit
Wang et al.’s podium abstract describes an evaluation of medical NLP libraries when applied to patient-reported adverse event reports.
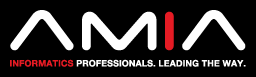
Symptomatic adverse events (AEs) such as nausea are common among patients enrolled in cancer clinical trials. Historically, this information has been collected and reported into research databases by clinical staff using a set of AE grading criteria maintained by the National Cancer Institute (NCI) called the Common Terminology Criteria for Adverse Events (CTCAE). In NCI’s Patient-Reported Outcomes version of CTCAE (PRO-CTCAE) software system, patients can also provide supplemental free-text narratives about their AEs. A majority of patients submit supplemental AE information when given the opportunity, and the supplemental data has little overlap with data reported through structured questionnaires. This means the data can be very valuable for understanding AEs. However, patient reported data is quite distinct in nature from the typical medical language used by clinicians or other formal sources of health information upon which typical medical NLP tools are trained.
To better understand the limitations of NLP tools for unstructured patient-reported AEs, we conducted a series of experiments comparing NLP performance against a set of manually labels produced by human review. The results highlight key limitations and opportunities for future work.
Yue Wang presented this work at the 2021 AMIA Informatics Summit. More details and a PDF of the extended abstract can be found on the publication’s page on this website.
Reference
Yue Wang, David Gotz, Ethan M. Basch, Arlene E. Chung (2021). An Evaluation of Clinical Natural Language Processing Systems to Extract Symptomatic Adverse Events from Patient-Authored Free-Text Narratives. American Medical Informatics Association (AMIA) Informatics Summit Podium Abstract.